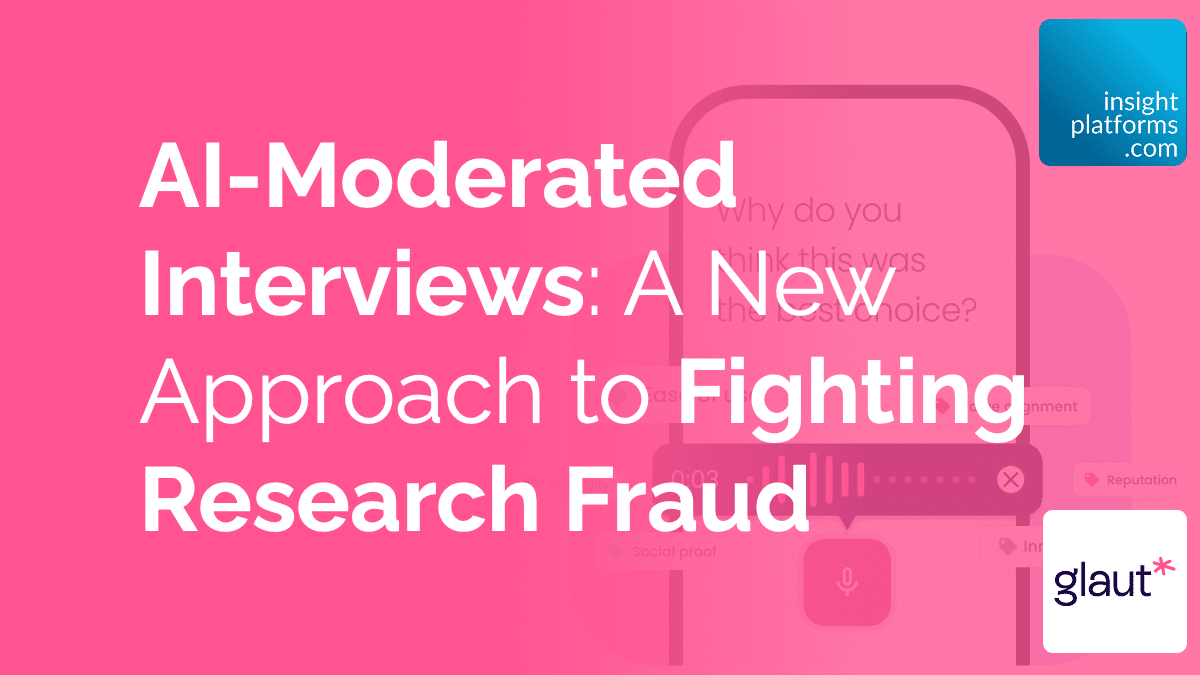
AI-Moderated Interviews: A New Approach to Fighting Research Fraud
By Insight Platforms
- article
- Qualitative Research
- AI
- AI Interviews
- AI Moderation
- Conversational AI
Poor data quality and fraudulent responses present important challenges in market research. However, as research methodologies evolve, new solutions emerge to address these issues. One such development is the AI-moderated interview (AIMI), a new approach that combines qualitative depth and quantitative efficiency while incorporating advanced fraud detection capabilities.
This article is based on Glaut’s webinar presentation. Watch the full demo replay here:
Glaut Lightning Demo: From Passive Detection to Active Defense: Leveraging AI-Moderated Voice Interviews for Real-Time Fraud Prevention
Stay up to date
Subscribe to receive the Research Tools Radar and essential email updates from Insight Platforms.
Your email subscriptions are subject to the Insight Platforms Site Terms and Privacy Policy.
What are AI-Moderated Interviews?
AIMIs occupy a unique position in the research methodology spectrum. They’re semi-structured, meaning there’s a framework guiding the conversation, but the AI moderator responds in real time, asking follow-up questions, probing for detail, and steering the discussion naturally. It’s essentially a hybrid between traditional surveys and one-on-one interviews.
One of the stand-out features is that they’re voice-based. Platforms like Glaut let respondents speak in their native language (over 50 supported), making the experience more natural and harder to manipulate.
The result? A method that scales like a survey but delivers richer insights, without the need for a human moderator.
Four Ways Glaut Uses AIMIs to Prevent Fraud
1. Voice-Only Mode
The voice-only mode represents a fundamental shift in data collection methodology as respondents can’t copy-paste, click through at speed, or use bots to spoof their way through the questions. Instead, they have to engage in real conversation. This system effectively eliminates several common forms of fraudulent behaviour.
2. Uncooperative Respondent Detection
This feature acts as a real-time safeguard that monitors participant behaviour throughout the interview process. The system automatically detects inattention, nonsensical inputs, and speeding. When uncooperative behaviour is identified, the system responds with additional probing questions. If the behaviour persists, the respondent is automatically removed from the interview, preventing low-quality data from entering the dataset.
3. Consistency Evaluation
The AI moderator employs smart memory capabilities to track responses across the interview. This allows it to spot inconsistent answers, flagging them for researcher review or automatically excluding them from the dataset, depending on the predetermined parameters.
4. Interpretative Scoring
This scoring system evaluates responses across three dimensions: clarity, specificity, and relevance. Each response receives a qualitative classification (high, medium, or low) that can be converted into numerical scores for analysis. Researchers can use these to identify high-quality responses and filter out lower-quality data before the reporting stage.
Who is It For?
AIMIs, and Glaut specifically, are designed for experienced researchers, whether in-house, at agencies, or as independent consultants. The platform supports a wide range of use cases, such as asset testing, customer experience evaluation, brand research, and other customisable research scenarios, including response piping, branching, quota management and screening.
Researchers can access Glaut’s technology through a do-it-yourself platform or with supported execution through field service teams.
Final Thoughts
AIMIs offer multiple layers of fraud prevention while maintaining data quality. Glaut’s combination of voice-based interaction, real-time monitoring, consistency checking, and interpretative scoring creates a robust system for collecting reliable research data.
The big difference? These quality control measures happen during the interview, not after. So researchers aren’t wasting time cleaning up data, instead, they are starting with cleaner, richer input from the beginning.
For anyone dealing with the headaches of dodgy data, AIMIs might just be the smarter way forward.