The Power of Automation and AI in Market Research
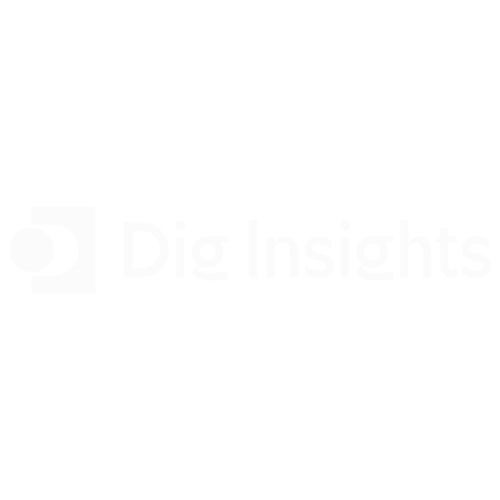
Discover how automation and AI tools can help you streamline and optimise the market research process.
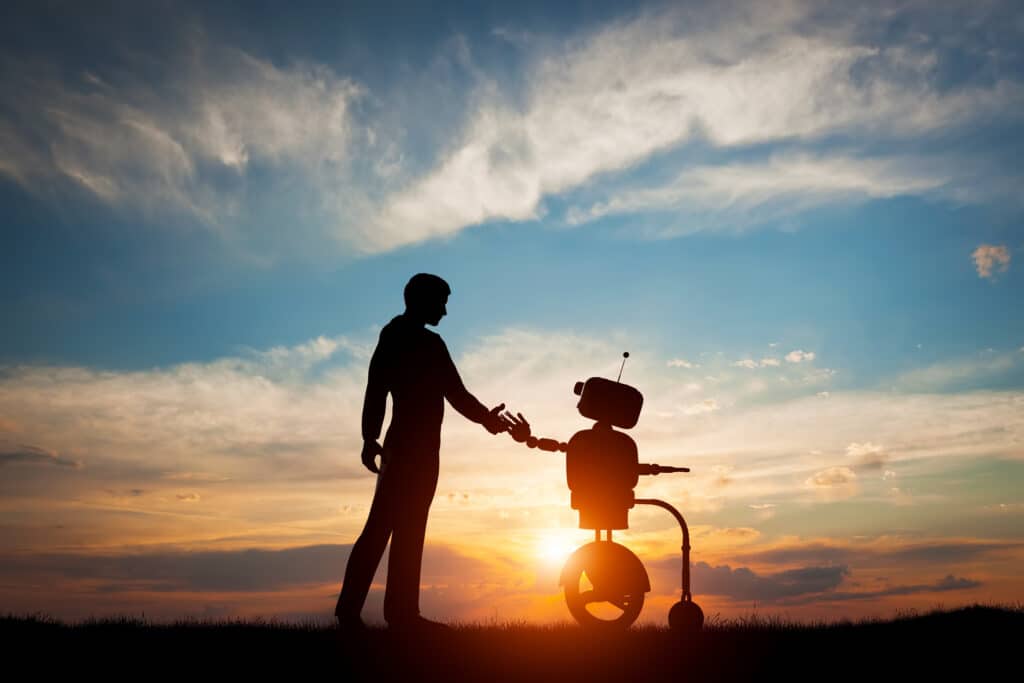
For the longest time, automation and Artificial Intelligence (AI) were only logical in science fiction. Even with the advent of computers, the logic and power behind them limited their capabilities. But as technology develops, rapidly increasing its potential and limiting its size, automation and AI applications in market research are becoming a reality.
- McKinsey predicted a complete shift in the job market because of automation by 2030.
- Forbes says that AI will make market research more accessible and affordable for businesses of all sizes
The pandemic was a huge accelerator of this change. As businesses were forced to shutter to keep their employees from getting sick, they also had to find ways to keep their business afloat, prompting them to invest in automation and AI wherever possible to help pandemic-proof their business.
With inflation and a lower unemployment rate creating an incredibly hot job market, it’s hard to find enough talent. Thus furthering the push toward automation and AI solutions wherever possible (remember ChatGPT). And that doesn’t even take into account the potential recession we’re facing, leading people and companies to tighten their purse strings.
All roads seem to lead to automation and AI. But they isn’t a one-size-fits-all, all-purpose, never faltering thing. So, how should you leverage them in your market research program?
First things first…
What is Automation?
Automation in market research refers to the use of technology to streamline and optimize various aspects of the research process. This can include automating data collection, analysis, and reporting, as well as using algorithms to identify patterns and insights in large datasets. By leveraging automation, researchers can save time, reduce costs, and improve the accuracy and reliability of their findings.
The Benefits of Insights Automation
Insights automation enables you to easily use platforms with a lot of functionality built into them. Templated approaches easily distill information. Data visualizations provide quick ways to prepare reports and provide insights to your stakeholders.
But what does that look like in practice?
Speeding Up Stage-Gate Innovation
The Stage-Gate process allows you to identify new ideas that should not be pursued before any serious financial commitment is made while also enabling you to provide resources to the viable ideas you want to develop. And automation dramatically improves the entire process.
Most large companies have distinct checks and balances that happen at each stage of product development, all the way from ideation to product launch. So many internal things must happen before launching a product. Automating certain aspects of that process allows you to speed up product development, getting through each stage faster. So, whether you’re testing initial product ideas or refining package claims before the launch, automating these results facilitates a quicker decision on the go/no-go element of the Stage-Gate process.
Mitigating Resource Scarcity
As recession predictions come in, resources are likely headed for a bit of a crunch. Scarcity of resources could mean less money, fewer people, or (the one thing you can never have enough of) time. Just from that mindset, it’s a good idea to try and take advantage of automation to help limit the impact of potential cuts caused by a recession.
Additionally, the information set you’re working with is becoming larger. Survey data, social listening, transactional data are all used to help map trends, understand consumers, and figure out strategy. We are constantly inundated with new ways to find more information to help better understand consumers. So, as people become a scarce resource, you won’t have enough time to distill all that information into actionable insights.
Distilling Information Sets
There is an overwhelming amount of information available to everyone across the business at any given moment. It’s no longer easy to compile, digest, and understand it.
It’s a standard human evolutionary trait. It started with leveraging math to help speed up our day-to-day. Then computers and computing power increased, enabling us to spend time on things deemed more important. And now, we’re starting to take advantage of artificial intelligence and machine learning to find insights that would have taken weeks to uncover. Now you can do it in days, hours, and possibly even minutes or seconds.
Automating Tactical Decision-Making
It’s much easier at the moment to automate tactical and execution-based survey research. It lends itself to making quick decisions for specific use-cases. What’s the claim I want to make about this product? Or put on this packaging? We know that product or feature innovation testing is a great way to quickly determine what makes sense for a new launch.
These insights are analyzed and displayed in easy-to-understand visualizations the moment a survey is complete so you can see which idea or claim makes the top of the list, saving you time from scouring data tables to determine your best option.
The Limitations of Insights Automation
Here’s the thing – you’re never going to automate strategy.
Foundational studies are essential to understanding your consumers and what motivates them. You might be able to automate some of the beginning phases of research by leveraging things like A.I. and machine learning, but you still need someone to make strategic decisions based on the insights.
A.I. can help you categorize and make sense of the data, but humans still have to know if it makes sense for your business, or if it’s even meaningful. Because insight is only as good as the decisions you can make because of it.
Once you’ve used insights to develop your business strategy, your go-to-market strategy, and your product development strategy, you can start automating those more tactical decisions we mentioned above.
How Should you Leverage Automation in Market Research?
How We Approached Automating Analytics
Dig Insights started focusing on automating repetitive tasks because our analytics team recognized they were answering similar questions regularly. It started out as making a simple script to free up time on the team’s end, and automation efforts have progressed from there. The first automated tool we built was in 2014. The year after that, we developed the first iteration of our TURF tool. And now, it’s evolved into building full tools for consultants or clients to use on their own, consisting of a correspondence tool, factor analysis, and network and MDS maps (a.k.a. multidimensional scaling), just to name a few.
At one end of the spectrum lies zero automation and zero democratization – one-off or highly customized requests. At the other end is a fully democratized, self-service platform that handles creation, fieldwork, and automated analysis of the results. (In our case, that’s Upsiide.)
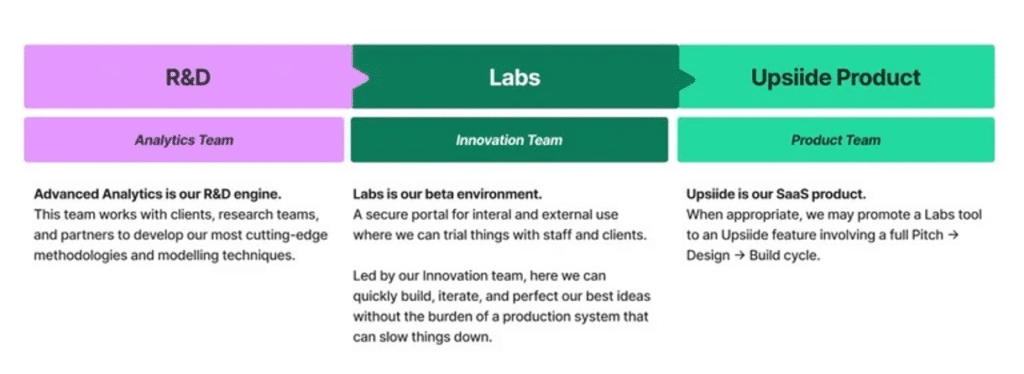
To make our way from 0 to 100, from no automation and democratization to full automation and democratization, we recognize when we get similar requests and start having to do something over and over.
Once we’ve got a good use case in mind, we put together a template within the analytics team that can ingest, transform, record, and fully analyze the data. From there, we build a script to simplify this process. But this is only semi-automated as it still requires some input – creating automated systems that always output correct data can be tricky. This is nowhere near being democratized.
After working out the kinks and ensuring we’re happy with the results (i.e., we don’t have to play around with the parameters for each test), we’ll make the script a fully automated tool. This now becomes a democratized tool for advanced analytics that our consultants can use.
From there, we’ll work towards transforming the tool into something anyone can use. Once it’s up to par, we work to integrate it into our Upsiide platform.
Respecting this process matters because if we can solve these problems once, we subsequently solve them for every study. Every study now reaps the benefits of this kind of standardized approach while still being customizable enough to handle any client need. Additionally, we can continue to work on improvements. For example, maybe we add another layer to a visualization tool to make it more interpretable.
But freeing up time isn’t the only area where you benefit from automation.
How We Automate Within DIY Reporting
A good example of how we took the complicated analysis of source of volume/share of choice and automated it is the introduction of our Market Simulator within Upsiide. We could automate this process because we controlled the collection of data and built algorithms specifically around that particular collection process.
Prior to building a self-serve source of volume simulator, our client experience team had to take the data collected and send it to our analytics team for analysis. Even though we already had an algorithm, the data sometimes needed formatting to enable successful analysis through our in-house algorithm. There are two potential problems here – human error and capacity.
When formatting data tables, there is always the opportunity for human error, which could lead to less accurate data. But, even if the data is formatted correctly, you still need someone to actually run the analysis. Waiting for an expert to do some advanced modeling, where you have to run models to get that data visualization, is something that might, in theory, only take a few hours. However, if everyone needs them, the bottleneck could make it take days.
However, because we’ve controlled the data and formatting with our new Market Simulator, we can transform the data the same way every time. Because we designed the data capture and built algorithms around it, a complicated methodology can be offered up on a DIY platform. You can now access an accurate market simulation in minutes.
We might be biased, but Market Simulator is a great example of finding a happy medium on the speed vs flexibility debate. It’s objectively fast (a report takes only a few minutes to run) and the modeling adapts to your use case seamlessly. It enables researchers and insights professionals to make a go/no-go decision on new products, flavors, and concepts.
How Should You Use AI in Market Research?
Quantitative Research
Generating New Ideas
AI means more than just analysis. When you push the boundaries, you can use AI for idea generation.
To create AI-generated ideas, you start with a large language model that understands natural language the way a human might. But this language model has read a curated version of half the internet, meaning it understands trends and preferences, so it can combine ideas across different categories to suggest new innovations automatically.
Innovation workshops are the perfect place for AI-generated ideas. Use AI to help you brainstorm. But only after you’ve interviewed relevant stakeholders to see what’s important. What direction do you want to go? What business plan/strategy does this align to? Are your consumers begging for you to be more socially conscious? Really anything that helps inform your innovation. Find your parameters and brainstorm a bit yourself. The more ideas you have and the more direction you can give the AI, the more likely it is to generate something completely new.
When testing our approach to AI with a client, our AI-generated ideas outperformed the majority of the ideas the client brought to the table, which goes to show that AI-generated ideas aren’t a fantasy but can actually help your innovation process.
And we have proof of that! We asked our team to use ChatGPT to come up with 20 brand-new milkshake flavor ideas and then tested them all on Upsiide. The idea was to see whose ideas would win out – those created by AI or those created by HI (human intelligence)?
ChatGPT did a great job creating creative flavors like “Blueberry Pancake Breakfast.” But humans thought about adding extra things into milkshakes like “Birthday Cake flavor with an actual cake slice on top.”
In the end, a human-generated idea won. The “Chocolate + Baileys” flavor came first with an Idea Score of 68 – just 1 point above “White Chocolate & Raspberry Truffle, which was an AI-generated idea.
Curious about the study?
Check it out on the platform here:
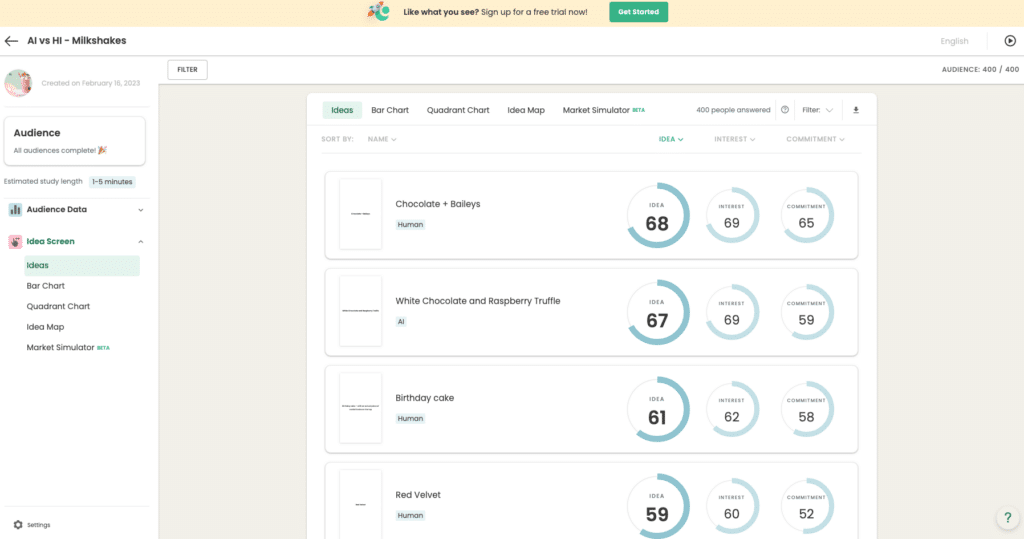
All this shows that AI can be a helpful tool to lean on in the early stages of innovation. Just make sure that you give the AI tool the right information to work with (e.g. examples and explanations), and it can deliver really creative ideas in a matter of seconds.
Collecting and Analysing Internal and External Data
The beauty of AI technology is that it’s collecting data all the time. And that’s what makes it such a powerful tool in market research.
By studying large amounts of data from various external sources, AI algorithms can identify patterns and trends that might not be immediately apparent to human researchers. This can lead to the discovery of new insights and opportunities that can help businesses stay ahead of the competition.
It’s especially handy when you’re working on a project for a long time. You kind of start developing bias towards certain ideas. For example, if you’re renovating a QSR menu, you might know from your previous experience that chicken burgers are usually successful with your target market. So, you subconsciously lean towards new types of chicken burgers you’re testing.
In that case, AI can act like the devil’s advocate. “Even though chicken burgers are great, I see a trend toward lamb meat among your target audience, so why not try lamb burgers?” Because it is in the business of data collection, AI allows you to think outside the box and consider new options.
Plus, if we think about the various stages of the innovation process (beyond idea generation), AI can help conduct predictive analytics. By analyzing past sales data and other relevant metrics, AI algorithms can predict future trends and consumer behavior. This information can be invaluable for businesses looking to introduce new products or change their marketing strategies.
Automating Repetitive Tasks
If you think about it, data isn’t that exciting. Few humans are eager to analyze large chunks of data – especially when we’re talking about survey data which contains heaps of unorganized responses. As a data or a research analyst, you might remember those long, boring Excel sheets that you have to go through to make a simple model or a report.
Well, an AI tool is more than happy to dig through that data for you. And it can do that much quicker (and probably more accurately) than you can. It’s an ideal partner in crime when it comes to repetitive tasks. And since you’ve saved time and money by automating this part of the innovation process, you can focus on the “bigger picture” and think about the strategy.
If you want to learn more about how you can use AI in market research, check out this episode of our Dig In podcast. Our EVP and in-house AI expert, Joel Anderson, talks about all the cool AI tools his team built and how he imagines the future of AI will unfold.
Qualitative Research
Quantifying Qualitative Feedback
We’re using AI to analyze open-ends through summarization or emotional sentiment tracking. Tools like Canvs AI are great partners of ours. Their tech helps us understand the emotional responses to open-ended questions in quant studies. A good example of how Canvs AI helps us quantify emotional responses is in our inflation report. Analyzing open-ends helped us understand there was (obviously) quite a lot of negativity surrounding how inflation affected consumers. But one thing we didn’t expect was the positivity people felt as a result of the economic downturn; they were proud of themselves for managing to cut back on non-essentials and create better budgets.
Moderating Conversations
Chatbots can be used in a few different ways in qual research – by becoming more of a moderator in a chat board, asking questions and following up with answers from the respondents. You can also use AI to prompt respondents to go deeper, avoiding the potential of receiving a blank open-ended question. Asking them to elaborate on why they said they didn’t like a product or offer.
For example, when trying to test new products to bring into the market, a respondent said they liked a spicy chicken sandwich. We then have an open-end that asks, “Why did you say you like the spicy chicken sandwich concept?” and the respondent says, “The bun looks yummy.” That doesn’t give us a lot of information about why they liked the new offering. So, the AI might chime in and say, “The bun looks yummy, but I want to know more about the sandwich! What kind of chicken is it? Is the spice level adjustable?”
This allows us to get more detailed information on what a respondent thinks about the products they’re reviewing.
Automation and AI in insights and market research is already here.
Automating or using AI tools for certain aspects of your insight generation will help you make decisions faster. But while this is true you still need to have a set strategy informed by good foundational research. Then you can execute and automate the more tactical research.
If you want a tool that helps you automate certain actionable insights like concept testing and claims testing, check out our Upsiide platform.
If you want more information on building strategies, don’t be afraid to reach out to us here.