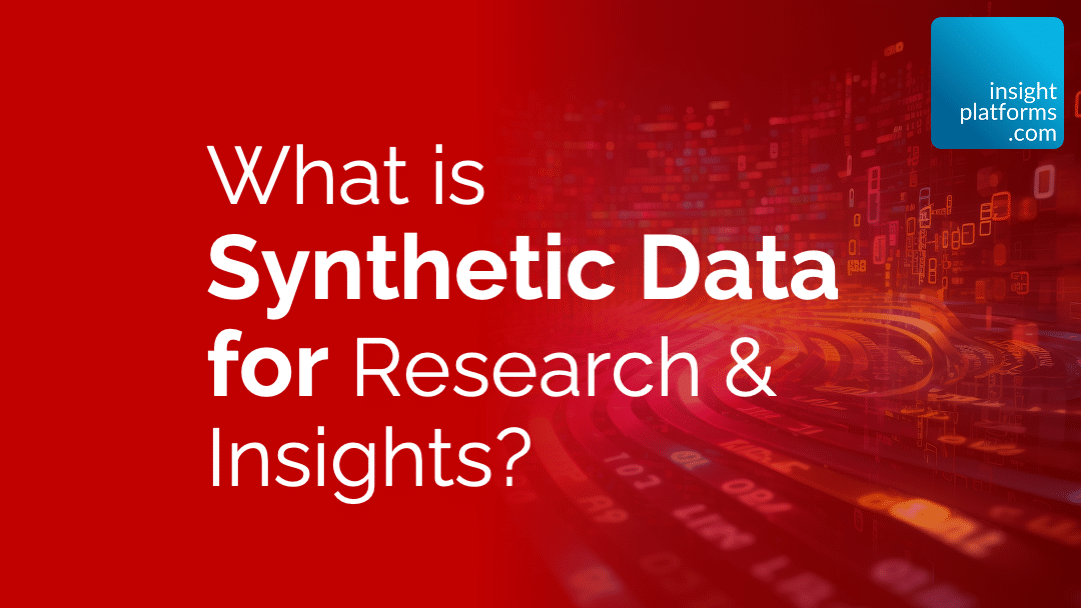
What is Synthetic Data for Research & Insights?
By Insight Platforms
- article
- Artificial Intelligence
- Synthetic Respondents
- Data Processing
- Statistical Analysis
- Statistics and Data Science
There is an undying need for more and more data in research. What if there was a way to replicate the insights of human data with artificial data to get even more of it? Synthetic data may be the answer.
Synthetic data is a form of artificial data that mirrors the complex patterns and nuances of real-world information without compromising individuals’ personal details.
After analyzing existing human datasets, AI-powered synthetic data generators are able to develop data that maintain the statistical properties of their “real” counterparts.
Synthetic data is entirely artificial and created by generative algorithms. These datasets are used for training machine learning models, enhancing data privacy, and conducting software testing – all without the risk of compromising anyone’s privacy.
Why Synthetic Data?
As collective consciousness around data privacy grows and regulations such as GDPR in Europe and CCPA in California have set new precedents for data protection, communities are rethinking their data strategies to shift towards synthetic data. Caution is an important practice as we enter an era when privacy and regulations are playing catch-up to digital and online advancements.
For instance, in healthcare, researchers can use synthetic patient records to improve medical research and treatments without endangering real patients’ privacy. By using synthetic data to develop artificial records, researchers can simulate various patient demographics, medical histories, and disease progressions without ethical concerns.
Synthetic data may also be used in software development or education, where users and students need data to work on projects without accessing sensitive or proprietary data.
Applications of Synthetic Data for Research & Insights
Synthetic data is increasingly being used in the development of software products and AI models.
Machine Learning and AI Model Training: Training AI models require enormous volumes of data – synthetic data can provide these volumes without the legal and ethical complexities of collecting and using real individuals’ data. The goal is to create more accurate AI models trained on diverse datasets that might be rare or unavailable in real-world data.
Customizable Software Testing: Synthetic data can be used in software development to simulate user interactions, network traffic, or security threats like cyberattacks, allowing developers to test applications under realistic conditions and identify potential performance issues before they occur.
But what are some of the big use cases for synthetic data in research & insights?
Research & Insights Use Cases
- Qualitative exploration: LLMs such as Synthetic Users can be prompted to generate user or consumer personas with relevant attributes (e.g., demographics, behaviors, product usage); these can then be interviewed to explore problems, brainstorm ideas, test concepts or understand journeys. This is particularly helpful for developing hypotheses or ‘pre-piloting’ primary qualitative research.
- Consumer Behavior & Product Testing: Yabble’s Virtual Audiences allows researchers to gather data on consumer preferences, shopper behavior across segments and even product concepts, features and packaging design.
- A/B Testing: With synthetic data, researchers can simulate user responses to two different configurations of a website, app, or other product features to determine which version provides the best user experience, hence optimizing conversion rates and user satisfaction.
- Privacy Protection in Sensitive Studies: In areas such as healthcare or the social sciences, synthetic data can represent population attributes while protecting individual identities, making it ideal for sensitive qualitative studies.
- Data Enrichment & Analysis: By blending synthetic data with real feedback data from surveys, researchers can enhance datasets, thus providing more robust material for product development or statistical analysis on datasets that are either too small, incomplete, or biased. Alternatively, in scenarios where collecting real data is challenging or costly, synthetic data serves as a feasible alternative, making it possible to conduct extensive quantitative research with fewer restrictions. Livepanel and Fairgen are two solutions for enriching primary data with synthetic data.
Generating Synthetic Data
The process of developing synthetic data is much more complex than just having AI copy and regurgitate “real-world” data.
Generating high-quality synthetic data is only possible with the use of effective algorithms. These algorithms must be able to produce data points that are both statistically and structurally identical to their real-life counterparts, without accidentally leaking actual user information or falling victim to overfitting (an error where a machine learning model performs well on training data but fails to make accurate predictions to new, unseen data).
There are two main tests used to determine the effectiveness and accuracy of synthetic data:
Comparing synthetic data against primary data collected from real-world observations can help researchers challenge benchmarks for utility, validity, and data accuracy.
Comparing synthetic data with real-world outcomes is great for evaluating the predictive power of a synthetic dataset. However, keep in mind that this method is challenged by the unpredictability of external factors that influence real-world events.
Depending on the information and the desired outcomes, there are several techniques utilized to generate synthetic data.
Rule-Based Generation: This method produces data using predefined rules and logic. It’s straightforward, making it ideal for creating structured data like test databases.
Data Augmentation: This involves expanding datasets by slightly altering existing data points or creating new ones based on existing data. This is an important strategy for fields where data is scarce or privacy concerns limit the use of real data.
Generative Adversarial Networks (GANs): By using two neural networks — the generator and the discriminator — GANs produce datasets that are extremely similar to genuine data, and they can even be used to generate datasets from images, videos, and audio.
The Controversy Around Synthetic Data in Research & Insights
The use of synthetic data and synthetic respondents in research has certainly sparked some controversy. The ethical considerations surrounding synthetic data are multifaceted, many argue that such algorithms cannot understand meaning and are only capable of making predictions based on the patterns they identify in their training data. There have also been concerns about the reliability of research findings based on synthetic respondent data, as well as the potential misuse or misinterpretation of synthetic data – leading to misleading conclusions or unethical practices.
Before diving head first into using synthetic data as a staple resource, it’s important users recognize the importance of continually testing the waters to monitor its capabilities and functionality.
Effectiveness and Applicability: How often should users monitor synthetic data to make sure it works as intended and under-identified conditions?
Bias Introduction: Do synthetic data algorithms introduce new biases into the research process, and how can users prevent these from potentially skewing results and causing harm when applied in real-world scenarios?
Timeliness: If synthetic data can become outdated, especially considering the decrease in primary data collection, how can users prevent stale or inaccurate representations?
Representation and Focus: With synthetic data gravitating towards mainstream societal segments, could this negatively impact underrepresented groups and worsen inequalities within data?
Transparency and Disclosure: How should synthetic data be disclosed to research buyers and users? Industry bodies like ESOMAR are starting to provide guidelines for the use of AI and synthetic data.
Despite all this, Gartner predicts that by 2030 most of the data used in AI will be synthetic data.
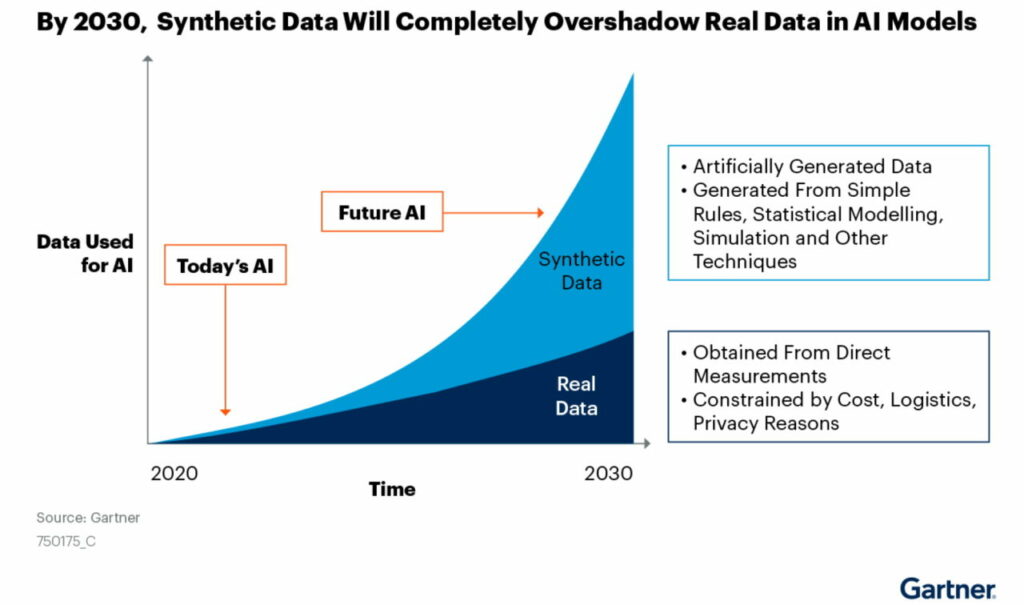
“The fact is you won’t be able to build high-quality, high-value AI models without synthetic data,” the report said.
The Future of Synthetic Data in Research & Insights
In healthcare, synthetic data is used to power research while upholding patient privacy. In finance, it generates insightful yet confidential transaction datasets. Urban planners use synthetic data to design and model city growth and infrastructure needs, and AI developers rely on it to train more accurate and robust models.
How will synthetic data impact the future of the research & insights industry? We are only at the beginning of the journey, but synthetic data has huge potential to be a pivotal component in the pursuit of innovation in an era of increased privacy.