A Gastrograph AI Case Study
A version of this article appeared earlier on the Gastropgraph AI blog.
Gastrograph AI is an artificial intelligence platform that models human sensory perception to predict consumer preferences, trends, and market performance of existing and new products.
Our SaaS products create real-time predictive decision metrics and insight at every stage of a product’s life-cycle from conception to consumption.
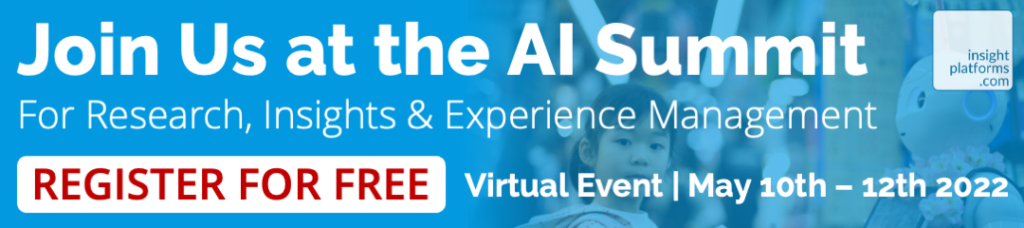
Located in Tokyo, Japan, the client company (“Client”) is one of the major fruit beverage manufacturers in Asia. The Client has been experiencing a continuous decline in sales in the last three quarters. The CTO, Mr. Saito, is seeking innovative solutions to improve the development cycle for the next new product release and turn the tide.
In addition, the company’s marketing team has suggested grapefruit, strawberry, and grape for the next development testing. The company currently uses traditional sensory panels for its new product development cycle. Each iteration takes about 5 months to complete.
The Problem
Mr. Saito and his team need new tools to understand consumer sensory perception and preference to develop new market-winning products for target demographics and cohorts. The Client’s marketing team has located three potential flavor solutions for the new product, but further validations need to be done in a more efficient and timely manner.
The Goal
The Client is looking for solutions to test and validate the currently proposed solutions and create new market-winning fruit-flavored beverages for active millennial females in Japan. Our goal is to understand the perception and preference of the target market, test and validate flavors requested by the Client in the target market, and explore new flavors with potential. Artificial intelligence is a new technology that can be utilized to better understand consumer sensory perception and preference of food and beverage products in the context of the market. Gastrograph AI uses this technology to help clients develop new competitive products and optimize existing brands.
The Solution
Utilize Gastrograph AI and take a 2-pronged approach to tackle the problems:
1. Competitive Market Survey
- Develops a benchmark for market performance based on flavor and texture profile for millennial females in Japan
- Shows both uncompetitive products and areas of low competition across flavors, preferences, and target demographics
2. Computational Creativity
- Explores new combinations of flavor that do not currently exist on the market
- Predicts the preference distribution of a target population for products without actual product formulation or sampling.
How does it work?
1. Competitive Market Survey
Understanding what consumers like or dislike about your products and competitors’ products is the very first step to developing a new market-winning beverage.
Competitive market survey analysis can be used to understand the flavor and preference space that the products under consideration inhabit. They can further be used to understand how much of the region has been explored versus unexplored, and where gaps can be filled. As there are multiple available methodologies that each highlight an aspect of the similarity or differences between products, we use two separate projections in this analysis, which are demonstrated below.
2. Flavor Profile Density and Preference
The following analysis visualizes the density of products with similar flavor profiles as a 2D map in order to show the location of select competitors within the class. As we can see in the graph, certain regions are extremely competitive, most of the products are highly concentrated, forming two major clusters in the flavor space, with only a few exceptions. This analysis can be used to locate clusters of products, determine the in-class contours of flavor, and identify white space. We observe a lot of flavor white space around the edges of the two major clusters, this offers a large blank canvas for Gastrograph AI to paint new flavors in regions of high consumer preference.

3. Flavor Profile Comparison Map
This projection shows each product mapped on a preference gradient on a multi-axis dimensionally reduced space. The variations between the products can be intuitively understood by comparing the locations of products to the origin of the graph and noting the direction of variance of its flavor attributes. This projection is best understood as a comparison between the flavor profiles of products and should be used to select products or regions that have a high preference or product groups that share similar signatures.
In this case, we can see the primary axis is separating variation of preferences around Fruits, Spices, and Sugars, which is a typical pattern for a fruit beverage product.

4. Computational Creativity
Gastrograph AI has the capability to explore new combinations of flavor that do not currently exist on the market; it predicts the preference distribution of a target population for products without actual product formulation or sampling.
Computational Creativity will iterate through many new flavor profiles to search for new combinations of flavor that will be most preferred by the target consumer demographic.
Initial Analysis
Current Product
The Client provides one of their current products for analysis. This is a lemon flavor beverage. The following graphs demonstrate the objective flavor profile (OFP) and the predicted preference distribution of the product in the target market.

Preference shows a bi-modal distribution, with an average Perceived Quality (PQ) score of 4.2.
- The distribution predicts about 17% of the consumers are going to dislike this product and give the product a PQ score of 3
- 25% of the consumers are going to like this product and give it a PQ score of 4
- 7% of the consumers are going to love this product, they are going to give the product a 7 out of 7.
Current Preference Distribution
The graph above shows the preference distribution of population in the target market across age and sex. In this specific case, we can see the product performs fairly well among 20-year-old women in the target population.
The overall performance of the current product is rather competitive. Clients will only accept any new products that perform at least as well as the current product.
Create a Blank Canvas
Gastrograph AI is able to create a “blank canvas” of the product for new flavor iterations.
This is done using an applied semantic vector, Gastrograph AI has specifically modeled how the perception of a variety of reference flavors, like ‘citrus,’ changes the resulting flavor profile and therefore the Perceived Quality scores for specific markets.
In the field of Natural Language Processing (NLP), linguists can perform executions like:
Taking a similar approach, Gastrographic AI is able to add and subtract different flavor signatures from a product’s flavor profile and predict the hedonic response (via the Perceived Quality metric) of the chosen consumer demographic.
This is a powerful theoretical approach to concept-testing the modification of any product by virtually testing different versions of the same product and predicting a consumer group’s response.

Validation: Three Flavors Suggested by the Marketing Team
AI in action
Let’s test the new flavors suggested by the Client’s marketing team.
1. Grapefruit

The performance of this flavor is worse than the original product. The red line represents the preference distribution of the new flavor, the blue line is the preference distribution of the original product.
As we can see, the predicted preference distribution has shifted to the left, this indicates that fewer consumers are going to like this product.
2. Strawberry

The performance of this flavor is even worse than grapefruit. The predicted preference distribution shifted more to the left.
This could be due to the highly competitive strawberry industry in Japan. Japanese consumers have been exposed to higher quality strawberry products that are readily available in the local market, thus crystallized into a higher preference standard towards strawberry as a flavor.
3. Grape

The performance of this flavor is still not satisfactory. The predicted preference distribution is out-performed by the original product. Actually, more people will give this product a 4 or lower.
The grape flavor is not the solution either.
Let the AI Create!
None of the flavors suggested by the marketing team surpass the performance of the original product.
It’s now urgent for Mr. Saito’s team to come up with new answers to the development team. We introduced Computational Creativity, a Deep Market Insights service, to Saito’s team.
Validation: Computational Creativity
AI in action
We need to set some basic boundaries for AI to generate new results. In this case, it’s for millennial females in Japan. We tuned the AI to be exploratory, meaning it is going to test new flavors including those that are generally uncommon to the target market.
Here is the result!
Pine

The Preferred Beverage Flavor for Millennial Females in Japan is Pine
The AI Predicts that over 40% of the target demographic is going to give this new pine flavored beverage a PQ of 6 or higher.

In fact, there is already some popular pine-flavored beverage that exists in the market. There is a well-known pine bud drink by Lotte in Korea (a strangely popular souvenir for tourists); there is a popular pine drink by Smirnoff in Europe; and in the United States, Deschutes has a pine flavored IPA.
Although the Client’s marketing team did not suggest pine as a new flavor, it does not mean that this flavor will not be preferred. In fact, Gastrograph AI is able to sense signals in the market, which implies potential pent-up demand in the target market.
Next Steps
The Gastograph AI team is going to work closely with the Client’s R&D team to hit the target flavor profile and develop the new product.
1) Levels of consumer preference for new product iteration for the target demographic will be carefully measured and reported to enhance the next iteration.
2) Prediction of preference evolution for the target demographic in the context of new product iteration will be delivered to ensure the Flavor and Texture Profile Optimizations are on the right track.
3) The new product is a complex mix of many different components, Gastrograph AI is going to profile the Flavor and Texture of the components (ingredients) in the new product.
4) The Gastrograph AI is going to deliver a blend optimization of multi-component products to ensure the product is going to hit the target flavor profile.