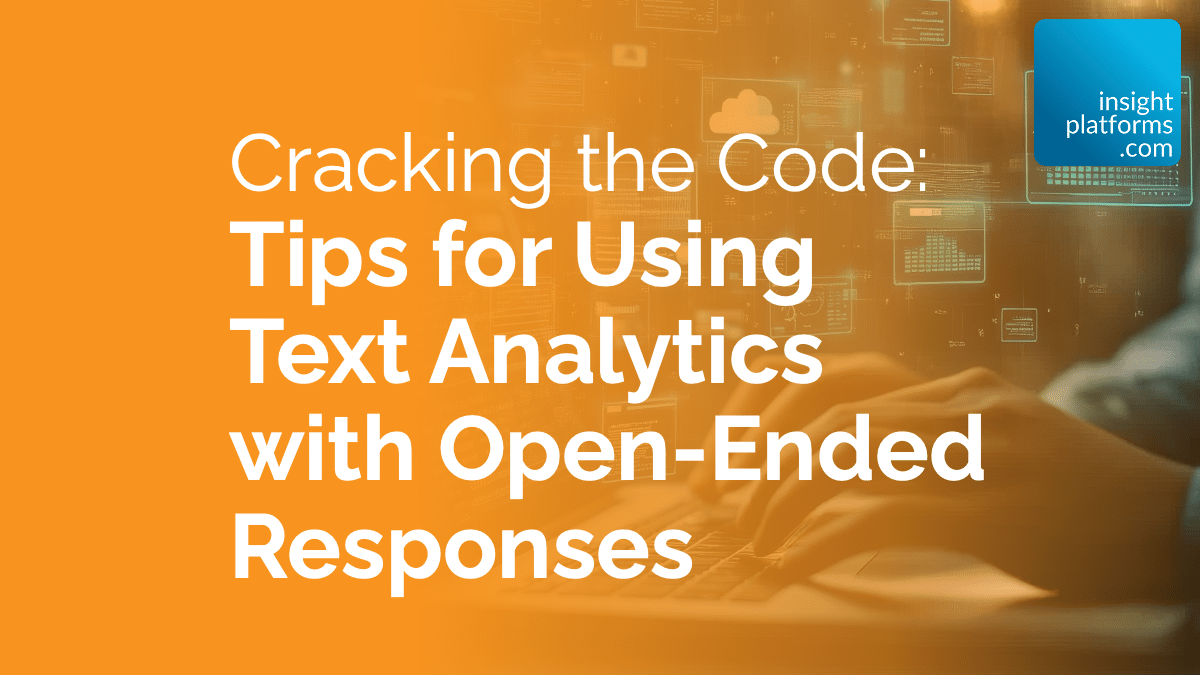
Cracking the Code: Tips for Using Text Analytics with Open-Ended Responses
By Insight Platforms
- article
- Text Analytics
- Automated Survey Research
- Survey Software
- Survey Research
- Survey Analysis
- Verbatim Response Coding
We all love open-ended questions to learn what respondents are really thinking. It produces real golden nuggets of insights but it can be quite painful to analyse mountains of unstructured data. This is where text analytics steps in: the use of structured coding organises this chaos, turning it into meaningful data. This process includes:
- Identifying and measuring trends and frequencies.
- Categorising responses into themes or groups.
- Measuring sentiment (positive, negative, or neutral).
The Top Tools for Text Analytics
I’ve seen countless demos and webinars showcasing the wonders of text analytics—like magic, tasks that used to take us hours of manual work are now done in mere seconds. And honestly? They’re right! The technology is impressive.
However, (you knew there was a “however” coming, right?) things can get a bit trickier depending on how you structure your survey, the tools used, the quality of responses, and so on. It doesn’t matter if you’re a seasoned researcher or a brave beginner, this is uncharted territory for many of us.
To make things even more fun, the technology keeps evolving even as you read this. I faced this very challenge while doing some research recently, and because we didn’t think things through, we made many mistakes from the beginning that led to a chaotic analysis using different tools.
With this experience in mind, I decided to put together these tips for success when diving into text analytics for open-ended responses.
Stay up to date
Subscribe to receive the Research Tools Radar and essential email updates from Insight Platforms.
Your email subscriptions are subject to the Insight Platforms Site Terms and Privacy Policy.
First Things First: Know Your End Game
Assuming you already did your homework and planned a beautiful and insightful survey, be responsible when creating open-ended questions and think them through beforehand.
Ask yourself, “What do I want to achieve with the question? What kind of results can I act on?”
Here are some examples of things you can do:
- Identify themes to understand strengths and weaknesses: group responses into categories like “delivery issues” or “product satisfaction.”
- Measure sentiment towards your brand and competitors: analyse whether responses are generally positive, negative, or neutral.
- Track keyword frequency for trends and opportunities: spot the most mentioned words or phrases.
It will be much easier to design the questions and choose what tools to use to analyse the outcomes when you understand what it is you are looking for.
Structure Your Questionnaire
A well-structured survey questionnaire is the backbone of any research, and especially for successful text analytics.
My tips for crafting effective questions:
- Be specific: Instead of vague prompts like, “What did you think?” ask something like, “What was the most memorable part of your experience with [X]?”. Leave nothing to imagination or you can get random words which won’t be relevant.
- Keep It Short and Sweet: Use concise, open-ended prompts like, “What could we improve about your experience?” rather than overwhelming people with compound questions.
- Avoid Open-Ends: Limit the number of open-ended questions unless absolutely necessary—your future self (and your text analytics tool) will thank you.
In our case, this was our first mistake. We didn’t want to prompt responses so we used only open-ended questions. Even though that was clear (at least) for us, we didn’t get the responses exactly as we wished.
For example, we structured open-ended questions like this, without any sort of pre-programming for the responses:
- 4.1. Which brand comes to mind first for yyy?
- 4.2. What words would use to describe ___?
- 4.3. Which brand comes to mind next for yyy?
- 4.4. What words would use to describe ___ ?
- 4.5. Which other brand(s) can you think of for yyy?
Even if this is not the ideal way to ask this question, it would have worked if people had only answered one brand for each question, or if they used only adjectives separated by commas, instead of complete sentences, or if they knew how to write the full name of the companies… and the list goes on.
That’s a lot of “ifs”. To get these insights, we would have needed a completely different structure:
- Use tools that capture the order of mentions
- Build the questionnaire to ensure the attribute is linked to the correct brand
- Add a list of pre-coded responses to the question – they can autocomplete or be used in the backend. And halfway through, add the new codes that appear to the list. It will help you organise mentions much faster.
Use the Right Tools
Not all text analytics tools are the same. The best tool for your project depends on your specific needs and budget. Here are a few popular options:
- AddMaple: Useful for simplifying qualitative data analysis.
- Displayr: A versatile tool for text analysis and visualisation.
- Codeit: Ideal for collaborative coding and managing large datasets.
- Caplena: Great for automated theme detection and categorisation.
- Fathom: Developed for coding and summarisation of unstructured text.
- inca AI coding: Designed for semantic labelling and analysing open ended responses.
- Canvs AI: Great for importing and analysing unstructured text from multiple sources.
Take some time to explore these tools and choose one that aligns with your workflow and expertise level. Also, they require different research and data processing knowledge to handle them, so adjust to what you can do as well.
As I mentioned, technology is awesome, but sometimes it can be a bit stubborn! Choose the tools before running the research – we did this afterwards and we ended up using 3 different platforms + good old Excel to get to grips with the data. I don’t recommend this – it is a nightmare to match the different datasets, styles and numbers and you will end up doing it all manually. Had we known which tool to use beforehand, we could have just built the questionnaire around it.
Most of these tools offer free demos. Try them! It might seem easier to watch a bunch of videos on their website, but they can be misleading by different datasets, easiness of pressing the button, upgrades on the tool that haven’t been considered, and so on.
Clean Your Data
Messy data leads to messy results. Before running your analysis, take the time to clean your dataset.
Steps to clean your data:
- Standardise formatting: Ensure consistent capitalization, spelling, and punctuation.
- Remove noise: Filter out irrelevant data, like “N/A” or incomplete responses.
- Correct typos: Tools may misinterpret common misspellings or slang.
Clean data is the foundation of accurate text analysis. This is part of the work that I recommend you not to leave all to the automation. It won’t work well, and you might end up with different rows for Cat, CAT or cat.
Balance Automation with Human Oversight
Automation is a lifesaver for categorising and analysing large datasets, but it’s not perfect. Tools can struggle with nuances like sarcasm, regional slang, or mixed sentiment. Try analysing social media mentions in Brazil – a sarcasm nightmare!
How to supervise the automation:
- Manually review part of the coded responses from several places of the data (not only the first records).
- Refine categories: Many tools allow you to customise themes and keywords—use this feature to align the analysis with your goals and make sure the themes and codes reflect what is inside them.
- Iterate as needed: If the results feel off, tweak your inputs, check the dataset for outliers or misplaced columns and run the analysis again.
Automation should be your assistant, not your replacement. It will do 70-80% of the grunt work for you – but your expertise and eyes are fundamental for great outcomes.
Pitfalls to Avoid
Even with the best tools and preparation, there are common mistakes to watch out for:
- Over-relying on automation: Tools can’t always pick up on context or nuance, they might not merge different ways of writing the same thing – make it simpler for the automation to do what it can and does best.
- Ignoring cultural differences: Language and sentiment can vary significantly across regions.
- Not planning the outcomes before the survey: Decide how you want to analyse results and tools before going into fieldwork.
- Skipping validation: Always sense check the results before distributing it.
- Over complicate the deliverables: Stick to one way of reporting and see the results – it is not worth it to use different tools because you want two ways of showing the same data.
Conclusion
Text analytics tools are powerful tools for coding open-ended responses, but they need a lot of balancing of technology with human expertise. It can turn raw feedback into actionable insights if planned well, with the right tools, and keeping a hands-on approach.
Always keep in mind it is not magic, and it is just a helpful hand to free you from grunt work and give you proper time to use your experience. It won’t replace human expertise and sense!