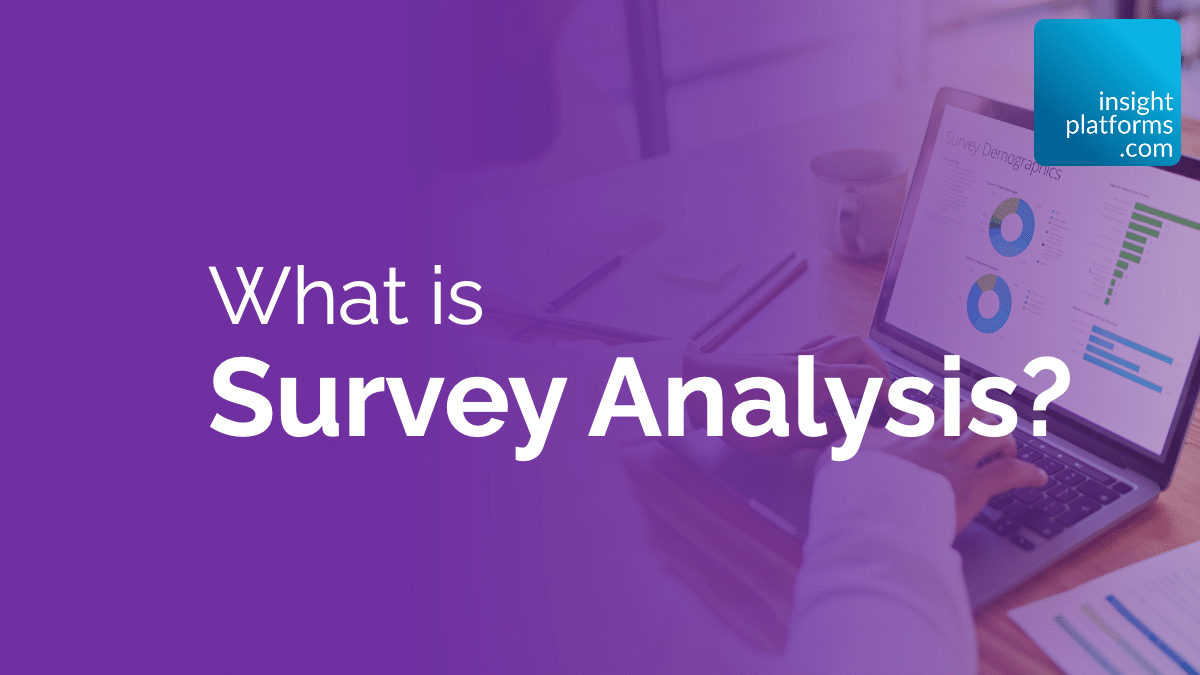
What is Survey Analysis?
By Insight Platforms
- explainer
- Survey Analysis
- Survey Software
- Cross-tabulation
- Statistical Analysis
- Statistics and Data Science
- Tabulation & Analysis
- Predictive Analytics
Survey Analysis is the process of turning the research data into actionable, business oriented insights. This article explains what survey analysis is and introduces some of the tools and methods to help you turn survey research data into relevant and actionable insights.
Not long ago, doing survey research was costly and needed a specialist agency. Then, technology made it possible for marketers and in-house researchers to conduct and analyse their own surveys – working in more agile ways.
One of the first widely accessible tools for online research and analysis, SurveyMonkey, now enables non-expert users to process and analyse results efficiently. However, the ability to gather results does not guarantee you will get the absolute best from your data: data on its own means nothing without good analysis.
Have a look at our top survey analysis tools or check out our introductory course if you’d like to learn more:
The Top Ten Survey Analysis Platforms
Essentials of Survey Design & Analysis
Types of Survey Analysis
There are a few ways to analyse data to respond to different types of business needs.
1. Descriptive Analytics
This is the most common approach and is one of the first ways to look at survey data.
It is relatively simple and describes the data captured. It could be used for quick answers but can also be the base for hypothesis creation – prior to statistical analysis – helping to identify outliers and patterns.
Common descriptive analysis approaches include:
- Mean, Median, Mode, Count, Percent, Frequency
- Range, Variance, Standard Deviation
- Percentile and Quartile ranks.
2. Inferential Analysis
This approach helps to preview future scenarios based on the analysis of past data.
It is recommended as a method of projecting the future behaviour of people, segments or markets. In addition, it allows you to evaluate economic fluctuations and consumption trends.
Usually based on long term survey results, this type of data mining uses several statistical tools to provide predictions:
- Correlations: evaluate the consequences of changes in specific variables.
- Cross-tabulation: analyse relationships between two or more variables.
- Regression: understand the relationship between a variable’s response to other variables responses, identifying direct impact of them.
This type of analysis can also make use of Machine Learning methods.
3. Diagnostic Analysis
This approach looks into the causes of an event, answering questions such as:
- Who?
- When?
- Where?
- How?
- Why?
It can be used as support and deep dive into descriptive analysis, as well as market and category insight generation.
4. Prescriptive Analysis
The most complex and with the highest added value, it is used to evaluate the consequences of actions taken, indicating the roadmap to achieve business goals.
It requires specific professionals and tools:
- Data Science tools (Big Data, Machine Learning, Artificial Intelligence etc.)
- Holistic business knowledge.
- Ecosystem deep knowledge.
Specialist analytics companies like Machine Vantage analyse ‘big’ data from many sources alongside survey results to identify patterns that could lead to new consumer behaviours.
A great example of how it worked for marketing purpose is the insight for new Ben & Jerry’s Breakfast flavours.
For truly insightful analysis, careful preparation is crucial – from from the brief, methodology choice, questionnaire development through to data collection. It’s important to know what you are looking for before you start collecting survey data.
Survey Analysis: A Practical Example
Good survey analysis goes beyond raw percentage answers (Descriptive Analysis) by transforming the data into insights and actions: finding the real story.
For example, if we are to evaluate customer satisfaction of attendees of an online course. We can simply ask them “Are you satisfied with the quality of this course?” and get an effective response:

It seems great, but there are still 18% unhappy customers, and we would not have any other information to help improving their experience.
Applying Survey Analysis techniques would bring us insights that could guide changes and improvement, driving these 18% customers to a happy place.
We could learn things like:
- Why are they not satisfied?
- Who are they?
- Who are the satisfied customers? (to compare)
In order to analyse survey results, the first step is to dig into the data and be able to combine information, analyse subgroups and apply your own knowledge of the topic.
Let’s look into ways to leverage survey analysis to a higher level, turning data into insights through statistics.
Cross Tabulation
Understand the relationship between 2 or more questions (variables). For example, we could cross the satisfaction answer against the profile of the attendee.

Filtering
Focus on a specific profile or subgroup, excluding the others. For example, looking into the Teachers’ group and how they have responded to other questions we can further understand their higher dissatisfaction.

Significance Testing
This helps you to understand whether any patterns in your data are likely to be genuine differences – or just the result of random variations.
Most survey analysis tools will identify whether differences between two numbers are statistically significant.
For example, if we say that teachers are significantly less satisfied than other groups at the 95% confidence level, it means that if we conduct the same survey 100 times, 95 times the results will be the same.
The confidence level depends on your sample size and how much the selected group represents the population you want to learn about.
Benchmarking
You can also compare your satisfaction results with competitors, for example.
You need to include them in your survey and gather responses from audiences that have attended classes in other platforms. Then it is possible to measure your performance within the market environment.

Trend Analysis
If you have previous research, you can also visualise how results have changed during a specific period, and measure the improvement of business performance.

Correlation
Understand how 2 or more variables move together.
For instance, we could look at the relationship between the level of seniority of professionals and their satisfaction. This might tell us that that the more senior they are, the less satisfied they are with the course – suggesting we need to improve course content for this audience.
Regression
More sophisticated analysis, it is used to understand impact of one or more independent variables on a dependent variable.
You can measure the impact of session times, topics covered, lecturers into the evaluation of satisfaction. Then it is possible to adjust what will make the most influence on satisfaction scores, instead of changing several bits of the course.
Tips for Successful Survey Analysis
Structuring the process of Survey Analysis will help you to reach deeper insights quicker and more effectively.
The following steps can make sure you get the most of it:
1. Identify the business question
Go back to the commercial questions driving the research.
These will be the top priority to focus on. Also, bear in mind that, more than one question combined could respond to the business issue.
2. Compare sub-groups
Are there any subgroups (age, gender, location, socio-demographic segment, product usage, brand knowledge etc.) within the sample?
If so, Filter your main questions by these groups to understand patterns and differentiation among them.
Even though some opportunities appear tiny in the Total Sample view, they could lead to niche (and valuable) opportunities.
3. Validate your hypothesis
Identify if the hypothesis designed from the beginning is real, or if there are new ones arising from the data.
4. Use statistical significance tests
Whenever possible and relevant, this is how you show results are trustworthy and representative.
For that, your sample must be big enough. These options should have been thought through during planning!
5. Stay focused on your priorities
Resist temptation to analyse every bit of data within every single subgroup available. This will add time, complexity and lose the interest of your audience.
Less is really more here: more compelling, more structured and far more relevant.
6. Visualise your results clearly
The correct graphic can do wonders to engage audience and create a friendly and informative report. Also, the right display can even intensify the point.
7. Bring context
Do not be afraid to branch out and bring external information (trends, previous research and surveys, public data etc.) that will contribute and base your findings and recommendations.
8. Give your point of view
Last, but not least, conclude.
Survey Analysis is not just about putting the results into a presentation. It is about extracting insights for the business strategy.
Make the main opportunities crystal clear in your storyline. It does not need to be a “conclusions” final page, it could even be within each chart, with the correct storytelling.
Pitfalls to Avoid in Survey Analysis
Very frequently researchers find themselves surrounded with loads of data, cross tables and analysis that never seems to end.
There is always another angle or breakdown that has not been covered yet.
So, the temptation is to keep digging, and the overall process can take more time and resources than you planned for.
The quality and relevance of the survey analysis depends on the analyst knowing when and where to stop digging.
Developing and analysing a survey is a continuous process with key focus points to ensure success:
- Have a clear and objective business issue or question to guide development of sample, research design, methodology and consequently, survey analysis.
- Pay attention to the quality of the questions to avoid bias, guiding responses or omitting options.
- Have a Data processing plan to ensure that the most relevant possibilities and cross tables will be possible.
- Focus the analysis over the main reason for the survey, to avoid over exploring.
When dealing with large amounts of data, the right survey analysis software is essential for analysing results. It will let you automate the process by analysing large amounts of data simultaneously.
There are several specialist tools available for Survey Analysis, including crunch.io, Infotools and Knowledgehound.
Some survey platforms have the analysis tools embedded, and you can even use tools like Microsoft Excel to calculate means, counts, percentages, or even filter information and correlate.
Finally, you may want to visualise your survey data in generic Business Intelligence platforms like Power BI and Tableau – but be careful. These software tools are not built specifically for survey analysis, and do not always let you structure your data in the right way.